What is this novel concept, and why does it matter?
This conceptual framework represents a novel approach to understanding complex systems, characterized by interconnected elements and dynamic interactions. It emphasizes the integration of disparate data sets and models, fostering a holistic perspective. This methodology aims to unveil hidden patterns and generate new knowledge through innovative analysis strategies. For instance, it might encompass utilizing computational models to predict outcomes in scenarios involving numerous interacting components.
The value of this approach lies in its potential to address multifaceted challenges across various domains, from environmental modeling to economic forecasting. By incorporating diverse perspectives and data sources, it promises to improve predictive accuracy and deepen our understanding of intricate systems. The historical context is marked by a growing need for integrative approaches to address global issues, and this framework is positioned to play a key role in achieving that goal.
The subsequent sections will delve into the specific applications and methodologies behind this conceptual framework, illustrating its utility in addressing contemporary problems. We will explore the practical implications of this approach and its capacity to transform existing paradigms.
berigalaxy
Understanding the multifaceted nature of berigalaxy requires exploring its constituent elements and the interrelationships between them. This framework provides crucial insights into its significance.
- Data integration
- Model synthesis
- Systems analysis
- Predictive modeling
- Complex interactions
- Holistic perspective
- Novel insights
- Interdisciplinary approach
These key aspects highlight berigalaxy's core function as a tool for comprehensive understanding. Data integration bridges disparate sources, enabling model synthesis to connect those sources into an overarching system. Analysis then reveals complex interactions within that system. The approach aims to yield predictive models and novel insights, requiring a holistic perspective and interdisciplinary collaboration. For example, modeling the effects of climate change on an ecosystem might utilize data from various sciences, building a complex model that forecasts interactions and potential outcomes.
1. Data Integration
Data integration is a fundamental component of the berigalaxy framework. Its significance stems from the inherent need to synthesize diverse datasets for a comprehensive understanding of complex systems. Without effectively merging information from various sources, a holistic view of the system in question is unattainable. This crucial step facilitates the development of more robust predictive models and insightful analysis.
- Data Fusion Techniques
The specific methods employed for merging data play a significant role in the effectiveness of the berigalaxy approach. Techniques such as data standardization, transformation, and reconciliation are essential for ensuring compatibility across different data sources. Common approaches include using ontologies to define common structures, employing data mapping to relate disparate formats, and utilizing data quality metrics to identify and resolve discrepancies.
- Handling Heterogeneous Data Sources
Real-world datasets often originate from various instruments, databases, and formats. Effective data integration within berigalaxy necessitates strategies for managing this heterogeneity. This includes employing appropriate tools and techniques for integrating data from disparate sources, such as relational databases, sensor networks, and textual data. Data cleaning, error detection, and consistency checks also become essential in such scenarios.
- Scalability and Efficiency
The volume and velocity of data in many contemporary contexts pose significant challenges to data integration. Solutions for scaling the integration process are essential. These include developing distributed data processing frameworks, employing cloud-based infrastructure, and implementing parallel processing algorithms. Efficient algorithms are vital for handling large datasets, ensuring timely integration, and enabling real-time analysis within the berigalaxy framework.
- Contextual Understanding
Integrating data is not merely about combining disparate datasets. A critical aspect of berigalaxy involves ensuring the integration process also accounts for the context and relationships within the data. This includes understanding the different meanings and interpretations associated with data from various sources, and incorporating that knowledge into the model. Examples of this include geographic location, time dependencies, or the relationships between various components within the system under study.
In summary, effective data integration is fundamental to the berigalaxy framework. The methodology requires robust strategies for handling heterogeneous data sources, ensuring scalability and efficiency, and maintaining contextual understanding to produce a holistic and accurate view of the complex systems under investigation. Without this critical component, the potential insights and predictive capabilities of the berigalaxy framework are significantly diminished.
2. Model Synthesis
Model synthesis, a crucial component of the berigalaxy framework, involves combining diverse models to construct a more comprehensive and accurate representation of a complex system. This integrative approach transcends the limitations of individual models, capturing the intricate interplay and emergent properties within the system. The inherent value of model synthesis lies in its ability to overcome limitations of individual models, offering a holistic understanding that single models cannot achieve. Real-world applications demonstrate the power of this approach. For instance, simulating climate change necessitates integrating models of atmospheric dynamics, ocean circulation, and terrestrial ecosystems, a process directly employing model synthesis. Similarly, understanding economic systems requires the simultaneous consideration of various market factors, encompassing consumer behavior, government policies, and international trade dynamicsall of which rely on model synthesis.
The significance of model synthesis within the berigalaxy framework cannot be overstated. It represents a fundamental step toward building predictive models that capture the intricate connections within complex systems. By effectively integrating disparate data and models, berigalaxy aims to not only identify existing patterns but also anticipate future developments. This is crucial for addressing contemporary challenges such as environmental sustainability, economic stability, and public health. By combining, for example, demographic models with epidemiological models, researchers can better forecast and prepare for outbreaks. This synthesis improves predictions in comparison to using only a single model. Further, a consolidated, multi-faceted model enables a deeper understanding of the system's behavior and underlying mechanisms, surpassing the limited insights provided by isolated models.
In conclusion, model synthesis is integral to the berigalaxy framework. Its power lies in its ability to combine diverse models, creating a more comprehensive understanding of complex systems. The process facilitates the development of predictive models that transcend the limitations of individual models, contributing to the advancement of problem-solving in various domains. This multifaceted approach is vital for tackling the intricate challenges of our increasingly interconnected world.
3. Systems Analysis
Systems analysis plays a critical role within the berigalaxy framework. It provides the methodological foundation for understanding the intricate relationships and interactions within complex systems. This analytical approach is essential for effectively interpreting integrated data and synthesized models, ultimately leading to a more comprehensive understanding and improved predictive capabilities.
- Decomposition and Modeling
Systems analysis relies on decomposing complex systems into manageable components. This process allows for a deeper investigation of individual elements and their interdependencies within the larger system. By identifying and modeling these interactions, researchers can gain insights into the emergent properties and behaviors of the entire system. For example, analyzing a supply chain involves breaking it down into segments of raw material sourcing, manufacturing, distribution, and retail, each with unique inputs and outputs.
- Identification of Key Variables
Systems analysis emphasizes the identification of crucial variables and parameters influencing the system's behavior. Careful consideration of these key factors allows for the development of targeted interventions and strategies. In environmental modeling, factors such as temperature, precipitation, and species interactions would be critical variables to isolate and assess.
- Feedback Loops and Dynamics
Recognizing and analyzing feedback loops and dynamic interactions within the system is vital. These loops represent cause-and-effect relationships where outputs influence inputs, potentially creating cyclical patterns and reinforcing behaviors. A thorough understanding of these dynamics is crucial for long-term predictions and effective interventions. For instance, understanding the feedback loop between resource consumption and population growth is critical for sustainable development.
- Data-Driven Validation and Refinement
Systems analysis methodologies employ data to validate and refine the model's accuracy. By comparing model predictions against observed system behavior, analysts can identify areas requiring modification and improve the model's predictive capabilities. This iterative refinement process is essential for maintaining the integrity and robustness of the model, making it suitable for more sophisticated insights and projections.
The methodologies of systems analysis are inherently integrated into the berigalaxy framework. The decomposition and modeling facilitated by systems analysis provide a structured approach to understanding the interactions within the complex systems modeled by berigalaxy. Identifying key variables and feedback loops enhances the predictive power of the integrated models. By iteratively refining these models based on empirical data, researchers achieve a more precise representation of the system's behavior. This rigorous approach ensures the reliability of berigalaxy's conclusions and the practicality of its applications.
4. Predictive modeling
Predictive modeling, a cornerstone of the berigalaxy framework, represents the capacity to forecast future trends and outcomes based on existing data and models. Its significance stems from the ability to anticipate potential scenarios and make informed decisions in complex systems. Within the berigalaxy context, predictive modeling leverages integrated datasets and synthesized models to project outcomes in multifaceted situations, enhancing preparedness and enabling proactive interventions.
- Data-Driven Forecasting
Central to predictive modeling is the utilization of historical data to develop statistical models or machine learning algorithms. These models identify patterns and relationships within the data, allowing extrapolation into the future. In berigalaxy, this data-driven approach leverages the integrated datasets, incorporating diverse variables and ensuring a comprehensive understanding of the system's behavior. For example, analyzing historical weather patterns, resource consumption, and economic indicators enhances the accuracy of climate change projections or macroeconomic forecasts.
- Model Refinement and Validation
The accuracy of predictive models is contingent on rigorous refinement and validation. Model performance is assessed against known outcomes and adjusted based on feedback. Within the berigalaxy framework, the integrated nature of models fosters a more robust validation process. Subsequent refinements based on the analysis of emerging patterns from data feed into the overall predictive capability, improving forecasts for future events. Consequently, models improve upon initial predictions and offer a more precise understanding of the system's dynamics.
- Scenario Analysis and Risk Assessment
Predictive modeling in the context of berigalaxy enables comprehensive scenario analysis. By utilizing various potential inputs and modeling diverse pathways, researchers can project multiple outcomes. This aspect is crucial in assessing risks and developing robust mitigation strategies. Such an approach is vital for forecasting and managing potential risks from various factors, including environmental change, economic instability, or public health crises.
- Adaptive and Iterative Modeling
Predictive modeling within berigalaxy is inherently iterative and adaptive. As new data becomes available or as the understanding of the system evolves, models are updated and refined. This continuous feedback loop ensures the models remain relevant and accurate, reflecting the dynamic nature of complex systems. This dynamic adaptability is a crucial element of berigalaxy, adapting to evolving conditions and refining future predictions.
In essence, predictive modeling within the berigalaxy framework is not a standalone tool but an integral part of a broader methodology for understanding and managing complex systems. By combining data-driven forecasting, model validation, and scenario analysis, berigalaxy enhances the accuracy and comprehensiveness of predictive capabilities, ultimately facilitating informed decision-making across numerous fields.
5. Complex Interactions
Complex interactions are central to the berigalaxy framework. The framework's core strength lies in its ability to model and analyze intricate, interwoven relationships within systems. These interactions are not merely additive; they create emergent properties and behaviors that individual components alone cannot predict. Consider an ecosystem: individual species interactionspredation, competition, symbiosisare complex; their aggregate impact on the entire ecosystem, influencing nutrient cycles and biodiversity, presents a higher-order complexity that the framework aims to capture. A similar principle applies to economic systems, where individual market behaviors create complex patterns of supply, demand, and price fluctuations. The intricate nature of these interactions demands a multi-faceted, systems-level approach that berigalaxy attempts to provide. Failure to account for these intricate interdependencies can lead to inaccurate predictions and ineffective interventions, highlighting the crucial importance of considering complex interactions.
Understanding complex interactions within berigalaxy necessitates examining the interactions among various elements of the system. This includes feedback loops, where actions of one component influence others, creating cyclical patterns. For example, an increase in consumer demand might stimulate production, leading to higher prices and subsequently reducing demand, illustrating a complex feedback mechanism. Additionally, berigalaxy models must acknowledge non-linear relationships where the effect of a change isn't proportional to the cause. A small change in one variable might trigger disproportionately large effects in others, making the system inherently unstable and difficult to predict, unless the entire system is modeled. Real-world examples abound: climate change, with its complex interplay of atmospheric, oceanic, and terrestrial systems, exemplifies the kind of system berigalaxy is designed to analyze. Another example is financial markets, where interactions between various actors and institutions influence market dynamics in unpredictable ways.
In summary, complex interactions are not merely a component of berigalaxy but its defining characteristic. The framework's strength lies in its capacity to model the intricate interplay of variables and processes. Ignoring these complex interdependencies leads to inaccurate representations of systems, hindering effective prediction and intervention. The need to comprehend and account for these complexities is crucial in addressing contemporary issues, such as environmental sustainability, economic stability, and public health, where understanding interconnected systems is paramount.
6. Holistic perspective
A holistic perspective is fundamental to the berigalaxy framework. It demands a comprehensive understanding of systems, encompassing not just individual components, but also the complex interplay between them. This approach recognizes that a system's behavior often emerges from the interactions of its constituent parts, exceeding the sum of its individual aspects. Adopting a holistic perspective is crucial for accurate predictions and effective interventions in complex systems.
- Interconnectedness of Elements
A holistic perspective emphasizes the interconnectedness of elements within a system. This interconnectedness is not simply a correlation; it involves recognizing how actions and changes in one part of the system ripple through other parts, creating feedback loops and cascading effects. Analyzing a supply chain, for example, requires understanding not only individual components like manufacturing, but also how changes in raw material availability impact downstream production, distribution, and ultimately consumer behavior. In berigalaxy, this interconnectedness informs the integration of data and models from diverse sources.
- Emergent Properties
Systems exhibit emergent properties that arise from the complex interplay of their components. These properties, often unpredictable from a study of isolated parts, are critical in understanding overall system behavior. An ecosystem, for instance, exhibits emergent properties such as biodiversity, resilience, and productivity, which are not simply the sum of the individual organisms, but rather arise from their complex relationships. Berigalaxy, by capturing these emergent properties, provides a more accurate representation of system behavior than models focusing only on individual components.
- Contextual Understanding
A holistic perspective recognizes that the behavior of a system is context-dependent. The same components acting in distinct environmental contexts might produce markedly different outcomes. Considering a city's infrastructure, the design of water supply systems needs to account for factors like topography, population density, and climate. A holistic approach examines how these elements influence the overall system's behavior and performance in berigalaxy's framework.
- Long-Term Impacts
A holistic perspective necessitates considering the long-term implications of actions and decisions. Short-term gains might have unforeseen long-term consequences in interconnected systems. In climate modeling, evaluating the long-term impacts of greenhouse gas emissions on weather patterns and ecosystems demands a holistic approach. This long-term view, deeply embedded in the berigalaxy framework, is essential for making well-informed decisions for complex systems.
In essence, the holistic perspective within the berigalaxy framework promotes a nuanced understanding of complex systems. By acknowledging interconnectedness, emergent properties, contextual factors, and long-term consequences, berigalaxy provides a more accurate representation and better prediction of system behavior. This fundamentally shifts the focus from isolated components to the intricate web of interactions that drives system outcomes.
7. Novel insights
Novel insights are a direct outcome of the berigalaxy framework. The framework's strength lies in its ability to integrate diverse data and models, generating a holistic perspective on complex systems. This integrated approach allows for the identification of patterns and relationships that might not be apparent through examining individual components in isolation. The synthesis of data from various sources, coupled with rigorous analysis, is precisely what fosters the emergence of novel insights. For instance, analyzing climate change using berigalaxy might reveal unexpected feedback loops between deforestation and atmospheric carbon concentrations, leading to a deeper understanding of the problem and potentially innovative solutions.
The generation of novel insights through berigalaxy has practical significance in numerous domains. In healthcare, identifying correlations between lifestyle factors and disease risk using berigalaxy can lead to personalized prevention strategies. In economics, novel insights might arise from understanding the interaction between monetary policy, global trade, and consumer behavior, potentially enhancing predictive capabilities and facilitating more effective policy decisions. Similarly, the analysis of urban development patterns using berigalaxy could reveal optimal urban design strategies that maximize resource efficiency and minimize environmental impact. These examples highlight the transformative potential of berigalaxy in uncovering new knowledge and actionable solutions across diverse fields.
In conclusion, novel insights are not a mere byproduct of berigalaxy; they are its primary outcome. By integrating diverse data sources and employing rigorous analysis, berigalaxy facilitates the discovery of previously unknown patterns and relationships within complex systems. These insights, in turn, are instrumental in addressing contemporary challenges and driving progress across multiple disciplines. The framework's ability to uncover and utilize novel insights is its key contribution to knowledge advancement and problem-solving in the modern world.
8. Interdisciplinary approach
The berigalaxy framework fundamentally relies on an interdisciplinary approach. This multifaceted perspective is essential because complex systems, the very subject of berigalaxy's analysis, often transcend disciplinary boundaries. Climate change, for example, requires understanding interactions between atmospheric science, oceanography, ecology, and economics. Similarly, analyzing the global financial crisis necessitates integrating insights from economics, sociology, and political science. An interdisciplinary approach is not merely a desirable add-on but a prerequisite for accurately representing and predicting the behavior of such systems.
The importance of an interdisciplinary approach in berigalaxy stems from the intricate nature of real-world problems. Isolated disciplines, focusing on specific aspects of a system, often fail to capture the interconnectedness that drives system-level outcomes. For instance, a purely economic model of resource depletion might overlook the ecological consequences, leading to inaccurate predictions. Conversely, an ecological model neglecting economic factors would miss crucial feedback loops that influence resource utilization. By integrating diverse perspectives and methodologies, the berigalaxy framework attempts to develop a comprehensive, more nuanced picture of the system's behavior. This holistic approach is key to generating actionable solutions for complex problems. Real-world examples like integrated assessments of sustainable development demonstrate the value of this interdisciplinary approach, as they acknowledge the interconnectedness of economic, environmental, and social factors. The approach is not merely additive; it unlocks novel insights that a single discipline might overlook.
In conclusion, the interdisciplinary approach is not just a characteristic of berigalaxy; it is its very foundation. By bringing together diverse disciplines, the framework strives to develop a more complete and accurate understanding of complex systems. This, in turn, enhances predictive capabilities and facilitates the development of effective solutions. While challenges remain in effectively integrating perspectives from various disciplines, the inherent value of this interdisciplinary approach is crucial in addressing contemporary issues, underscoring the necessity of collaborative research to solve complex problems in the 21st century.
Frequently Asked Questions (Berigalaxy)
This section addresses common inquiries about the berigalaxy framework. These questions and answers aim to provide clarity and context for understanding the framework's principles, applications, and limitations.
Question 1: What is the core objective of the berigalaxy framework?
The berigalaxy framework aims to provide a holistic understanding of complex systems. It seeks to integrate diverse data sources and models, facilitating a more complete representation of system dynamics than traditional, isolated approaches. This integrated analysis enables the development of more accurate predictive models and a deeper comprehension of complex interactions within a system.
Question 2: How does berigalaxy integrate data from different sources?
The framework employs various data fusion techniques. These include standardizing data formats, harmonizing units of measurement, and resolving discrepancies. Ontologies and data mappings are frequently used to establish connections between disparate datasets. The critical aspect of this integration is not just combining data, but understanding the context and relationships within the data to achieve a unified view of the system.
Question 3: What distinguishes berigalaxy from other modeling approaches?
Berigalaxy distinguishes itself through its holistic and interdisciplinary perspective. Unlike models relying on isolated datasets or single disciplines, it integrates diverse data sources and methodologies, accounting for complex interactions and emergent properties. This integrated approach allows for a more comprehensive representation of the system's behavior, leading to more nuanced and potentially more accurate predictions.
Question 4: What are the potential applications of berigalaxy?
Berigalaxy finds applications in numerous fields including environmental modeling (e.g., climate change), economic forecasting (e.g., market fluctuations), and public health (e.g., disease outbreaks). By providing a structured approach to analyzing complex systems, berigalaxy can improve predictive capabilities and facilitate informed decision-making.
Question 5: What are the limitations of the berigalaxy framework?
The computational complexity of integrating diverse datasets and models can be substantial. Data quality and consistency across sources are essential but can be challenging to achieve in real-world applications. The accuracy of predictions hinges critically on the quality of integrated data and the appropriateness of the chosen models, and this limits the precision of predictions in complex systems. Further, unforeseen interactions between components of the complex systems under analysis can confound the accuracy of predictions. Nevertheless, advancements in computational power and data integration techniques will continue to enhance the framework's applicability and minimize these limitations.
Key takeaways include berigalaxy's potential to provide comprehensive insights into complex systems, its interdisciplinary approach, and the importance of data quality and model selection. Future considerations and advancements can enhance its robustness and accuracy.
The subsequent section will delve into the practical implementation of the berigalaxy framework, demonstrating its application in various real-world scenarios.
Conclusion
This exploration of the berigalaxy framework has highlighted its multifaceted nature. The core tenets, encompassing data integration, model synthesis, systems analysis, and predictive modeling, form a powerful approach to understanding complex systems. A key strength lies in its ability to move beyond isolated disciplinary perspectives, fostering an interdisciplinary, holistic understanding that is vital for addressing multifaceted global challenges. The framework's emphasis on complex interactions and emergent properties provides a deeper, more nuanced analysis than traditional approaches. Furthermore, berigalaxy's capacity for generating novel insights, identifying previously unconsidered relationships within systems, holds significant potential for advancing knowledge and driving progress across numerous fields. A critical aspect underlined throughout the analysis is the imperative for rigorous data quality and model validation in ensuring the framework's accuracy and applicability.
The future trajectory of berigalaxy hinges on advancements in computational power, data integration techniques, and interdisciplinary collaboration. Further research is needed to refine the framework's application to specific contexts, addressing the complexities of real-world scenarios. The potential for berigalaxy to enhance predictive capabilities in areas such as climate modeling, economic forecasting, and public health is substantial, offering valuable tools for informed decision-making and proactive intervention. Ultimately, the widespread adoption and refinement of berigalaxy can contribute significantly to a more comprehensive understanding of complex systems and facilitate effective solutions to global challenges.
You Might Also Like
5Movierulz 2023 Download - Latest Movies!Corey Harrison: Unveiling His Impactful Journey
Foreigner Seeking Love: Understanding Love's Meaning
Lyracr0w0: Latest Tweets & More!
Anna Malygon: Latest News & Updates
Article Recommendations
- Inspiring Life And Impact Of Cana Norton
- Ultimate Guide Austin Powers Series Order Amp Insights
- The Art And Culture Of La Gastronomia A Culinary Celebration

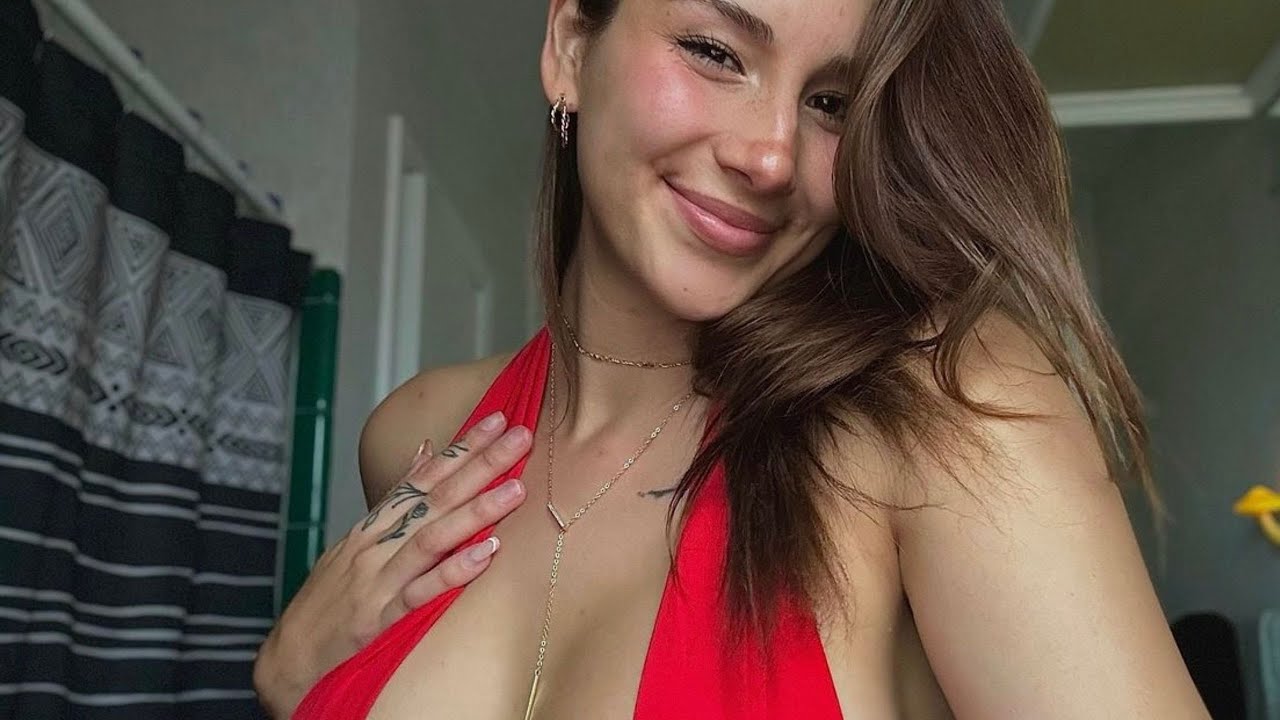
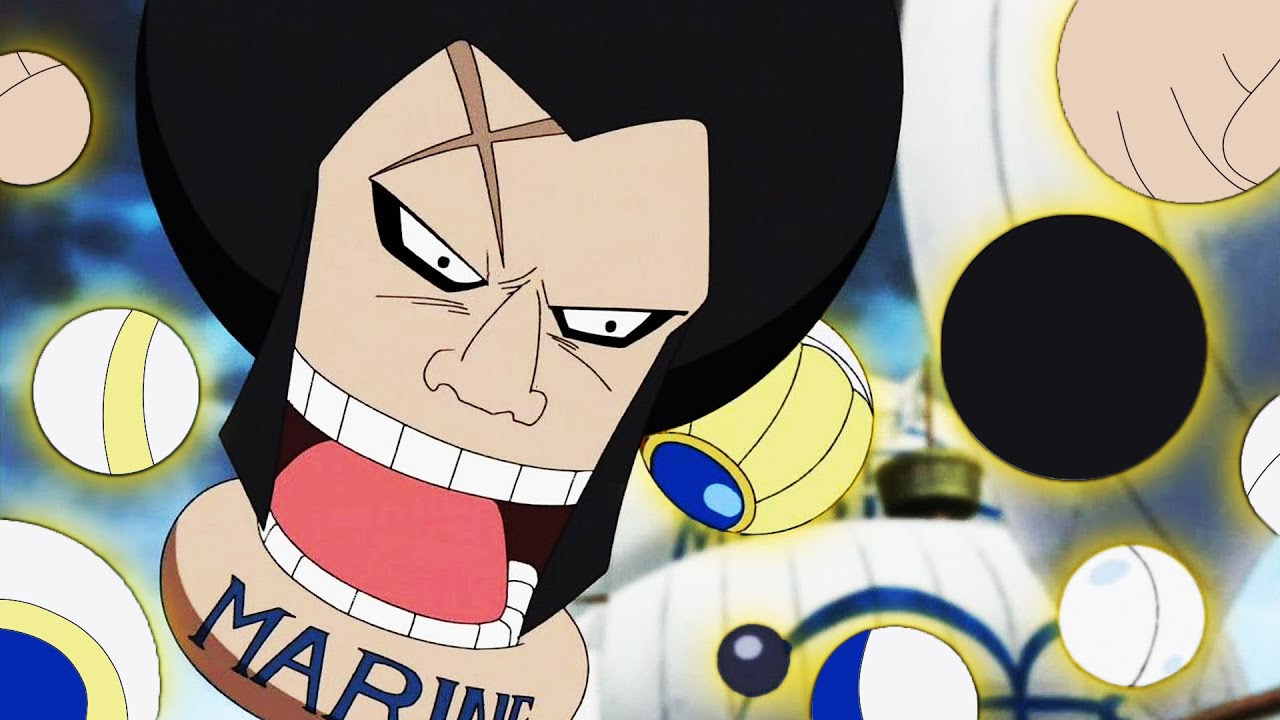